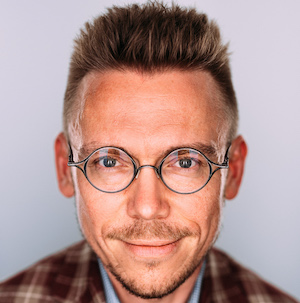
Jozef Barunik
About
Jozef Baruník is an Associate Professor at the Institute of Economic Studies, Charles University in Prague, where he is the Director of the Master in Finance and Data Analytics (MFDA) programme. He is also head of the Econometrics Department at the Czech Academy of Sciences. In his research he develops mathematical models for understanding financial problems (such as the measurement and management of financial risk), develops statistical methods and analyses financial data. He is particularly interested in asset pricing, high-frequency data, financial econometrics, machine learning, high-dimensional financial datasets (big data), and frequency domain econometrics (cyclical properties and behaviour of economic variables).
In addition to his academic roles, Jozef has contributed to the practical application of his research, and often takes on a variety of roles such as panel member for ERC consolidator, an external consultant for the Market Intelligence division of the Bank of England or other funding agencies and professional bodies.
Jozef’s research has appeared in the Review of Economics and Statistics, Econometrics Journal, Journal of Financial Econometrics, Journal of Financial Markets, Econometric Reviews, Journal of Economic Dynamics and Control, The Energy Journal, he is an associate editor of the Digital Finance, Journal of Economic Interaction and Coordination, and Kybernetika.
Submitted Publications
The Dynamic Persistence of Economic Shocks (with L.Vacha) preprint draft (June 2023)
Revise and resubmit, Review of Economics and Statistics
replication code and package in Julia
Common Idiosyncratic Quantile Risk (with M.Nevrla) preprint draft (Oct 2024)
Learning Probability Distributions of Day-Ahead Electricity Prices (with L.Hanus) preprint draft (Oct 2023)
Revise and resubmit, Energy Economics
code and package in Julia
Predicting the distributions of stock returns around the globe in the era of big data and learning (with M.Hronec and O.Tobek) (August 2024)
Revise and resubmit, International Journal of Forecasting
Deep Learning, Predictability, and Optimal Portfolio Returns (with M.Babiak)
preprint draft (July 2021)
Selected Publications
Predicting the volatility of major energy commodity prices: the dynamic persistence model (with L.Vacha)
Energy Economics (2024), Vol. 140, 107982
replication code and package in Julia
Dynamic industry uncertainty networks and the business cycle (with M.Bevilacqua and R.Faff)
Journal of Economic Dynamics & Control (2024), Vol. 1598, 104793
Persistence in Financial Connectedness and Systemic Risk (with M.Ellington)
European Journal of Operational Research (2024), forthcoming
code and package in Julia and Matlab
Quantile Spectral Beta: A Tale of Tail Risks, Investment Horizons, and Asset Prices (with M.Nevrla)
Journal of Financial Econometrics (2023), Vol. 21, No. 5, pp. 1590–1646
replication codes
Asymmetric Network Connectedness of Fears (with M.Bevilacqua, and R.Tunaru)
The Review of Economics and Statistics (2022), 104(6): 1-13
replication codes and data
Measurement of Common Risks in Tails: A Panel Quantile Regression Model for Financial Returns (with F.Cech)
Journal of Financial Markets (2021), 52, 100562,
replication codes and data
Forecasting dynamic return distributions based on ordered binary choice (with S.Anatolyev)
International Journal of Forecasting (2019), 35(3), pp.823-835,
code and package
Quantile Coherency: A General Measure for Dependence between Cyclical Economic Variables (with T.Kley)
The Econometrics Journal (2019), 22(2), pp. 131-152,
code and package
Supplementary material available
Total, asymmetric and frequency connectedness between oil and forex markets (with E. Kocenda)
The Energy Journal (2019),vol 40, pp. 157 - 174,
codes for introduced measures
Note the package frequencyConnectedness available here can be used to replicate the paper
Supplementary material available
Measuring the Frequency Dynamics of Financial Connectedness and Systemic Risk (with T.Krehlik)
Journal of Financial Econometrics (2018), 16 (2), pp. 271–296,
code and package
Supplementary material available
Do co-jumps impact correlations in currency markets? (with L.Vacha)
Journal of Financial Markets (2018), 37, pp.97-119,
codes for introduced measures
Modeling and Forecasting Persistent Financial Durations (with F.Zikes and N.Shenai)
Econometric Reviews (2017), 36:10, 1081-1110,
Supplementary material available
Estimation of financial agent-based models with simulated maximum likelihood (with J.Kukacka)
Journal of Economic Dynamics and Control (2017), 85, pp. 21-45,
Asymmetric volatility connectedness on forex markets (with E. Kocenda and L.Vacha)
Journal of International Money and Finance (2017), 77C, pp. 39-56,
code for replication
Cyclical properties of supply-side and demand-side shocks in oil-based commodity markets (with T.Krehlik)
Energy Economics (2017), 65, pp.208-218,
code and package codes for bootstrap
Good volatility, bad volatility: Which drives the asymmetric connectedness of Australian electricity markets? (with N. Apergis and M.Lau)
Energy Economics (2017), 66, pp.108- 115,
codes for introduced measures
Note the package frequencyConnectedness available here can be used to replicate the paper
Asymmetric connectedness on the U.S. stock market: Bad and good volatility spillovers (with E. Kocenda and L.Vacha)
Journal of Financial Markets (2016), 27, 55–78,
codes for introduced measures
Note the package frequencyConnectedness available here can be used to replicate the paper
Semiparametric Conditional Quantile Models for Financial Returns and Realized Volatility (with F.Zikes)
Journal of Financial Econometrics (2016), 14 (1), 185–226,
Supplementary material available
Forecasting the term structure of crude oil futures prices with neural networks (with B.Malinska)
Applied Energy (2016), 164, pp.366–379,
Modeling and forecasting exchange rate volatility in time-frequency domain (with T.Krehlik and L.Vacha)
European Journal of Operational Research (2016), 251 (1), pp. 329–340,
codes to paper
Volatility spillovers across petroleum markets (with E. Kocenda and L.Vacha)
The Energy Journal (2015), 36(3), 309-329
codes for introduced measures
Note the package frequencyConnectedness available here can be used to replicate the paper
Are benefits from oil - stocks diversification gone? A new evidence from a dynamic copulas and high frequency data (with K.Avdulaj)
Energy Economics (2015), 51, pp. 31-44,
Realizing stock market crashes: stochastic cusp catastrophe model of returns under time-varying volatility (with J.Kukacka)
Quantitative Finance (2015), 15 (8), pp. 1347-1364,
Realized wavelet-based estimation of integrated variance and jumps in the presence of noise (with L.Vacha)
Quantitative Finance (2015), 15 (6), pp. 959-973,
codes for introduced measures
Are Bayesian Fan Charts Useful? The Effect of Zero Lower Bound and Evaluation of Financial Stability Stress Tests (with M.Franta, R.Horvath,K.Smidkova)
International Journal of Central Banking (2014), 10(1), 159-187
Comovement of energy commodities revisited: Evidence from wavelet coherence analysis (with L.Vacha)
Energy Economics (2012), 34(1), pp. 241–247,
Can a stochastic cusp catastrophe model explain stock market crashes? (with M.Vosvrda)
Journal of Economic Dynamics and Control (2012), 33(10), 1824-1836
Full list of publications available here
Working Papers (Work in Progress)
Quantile Preferences in Portfolio Choice: A Q-DRL 1 Approach to Dynamic Diversification (with A.Sarkany and L.Janasek) (May 2024)
Abstract: We develop a novel approach to understand the dynamic diversification of decision makers with quantile preferences. Due to unavailability of analytical solutions to such complex problems, we suggest to approximate the behavior of agents with a Quantile Deep Reinforcement Learning (Q-DRL) algorithm. The research will provide a new level of understanding the behavior of economic agents with respect to preferences, captured by quantiles, without assuming a specific utility function or distribution of returns. Furthermore, we are challenging the traditional diversification methods as they proved to be insufficient due to heightened correlations and similar risk features between asset classes, and rather the research delves into risk factor investing as a solution and portfolio optimization based on them.
“Who’s the Boss?”” The Role of Shareholders in Banks’ Lending Decisions (with P.Katsoulis, E.Gerba and J.A. Smith)
preprint draft (Oct 2024)
Abstract: We investigate banks’ lending decisions in the face of shareholder pressure to make payouts. We exploit the regulatory ban on banks’ distributions during the Covid-19 pandemic to show that the conflict between the interests of banks’ shareholders and their borrowers can persist even with such a ban. First, we find that restricted banks faced an increase in shareholders’ required rate of return, which was even higher for the banks most exposed to income-oriented investors. This led to an increase in the required rate of return on their overall capital. Second, banks responded by increasing lending volumes only to medium-sized and larger firms and on smaller loans, which are less capital intensive. However, they utilised government guarantee schemes to increase lending volumes to smaller firms and on larger loans. The results suggest that distribution restrictions can incentivise banks to increase lending, but in a way that minimises the capital impact to appease shareholders.
Common Firm-level Investor Fears: Evidence from Equity Options (with M.Bevilacqua and M.Ellington)
preprint draft (Sept 2023)
Abstract: We identify a new type of risk, common firm-level investor fears, from commonalities within the cross-sectional distribution of individual stock options. We define firm-level fears that link with upward price movements as good fears, and those relating to downward price movements as bad fears. Such information is different to market fears that we extract from index options. Stocks with high sensitivities to common firm-level investor fears earn lower returns, with investors demanding a higher compensation for exposure to common bad fears relative to common good fears. Risk premium estimates for common bad fears range from -5.63% to -4.92% per annum.
Currency Network Risk (with M.Babiak)
preprint draft (May 2023)
Presented at at 2023 WFA (San Francisco), 2023 SGF Conference (Zurich), 2023 EEA-ESEM (Barcelona)
Abstract: This paper identifies a new currency risk stemming from linkages between option-implied currency volatilities. A volatility network strategy that buys net recipients and sells net transmitters of transitory shocks to ex-ante currency volatilities generates significant excess returns. Net recipients are more exposed to volatility spillovers and compensate investors with higher average returns. In contrast, net transmitters are more resilient to volatility transmissions and offer a lower risk premium because they hedge against volatility interdependencies. When volatility linkages are controlled for contemporaneous correlations, the strategy is uncorrelated with popular benchmarks. The volatility network factor is also priced in a currency cross-section.
The video below illustrates the short-term currency network during the Global Financial Crisis. If the video does not play properly, feel free to watch it here.
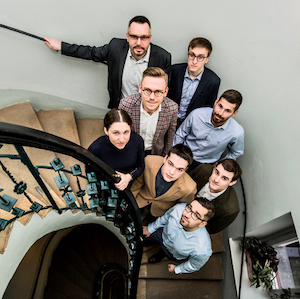
Students
Current (Full-Time) Doctoral Students
Attila Sarkany: Machine Learning in Finance
Lukas Janasek: Quantile Deep Reinforcement Learning in Economics
Josef Kurka: Horizon-specific risks, higher moments, and asset prices
Martin Hronec: Asset Pricing with Quantile Machine Learning
Lenka Nechvatalova: Deep Reinforcement Learning and Portfolio Management
Past Doctoral Students
Matej Nevrla: Tail risks, asset prices, and investment horizons
Lubos Hanus (joint supervision with L.Vacha) Dynamic density forecasting using machine learning
Krenar Avdulaj: Essays in Financial Econometrics
Frantisek Cech: Three Essays on Risk Modelling and Empirical Asset Pricing
Tomas Krehlik: Applications of Modern Spectral Tools in Financial Econometrics
Jiri Kukacka: Estimation of Financial Agent-Based Models
Barbora Gregor: Three Essays on Data-Driven Methods in Asset Pricing and Forecasting
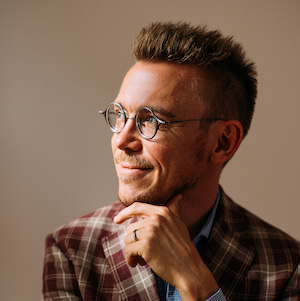
Teaching
JEM217 - Advanced Econometrics (Masters)
The objective of the course is to help students understand several important modern techniques in econometrics and apply them in empirical research and practical applications. Emphasis of the course will be placed on understanding the essentials underlying the core techniques, and developing the ability to relate the methods to important issues faced by a practicioner.
By completing this course, students will be able to use a computer based statistical software to analyze the data, choose appropriate models and estimators for given economic application, understand and interpret the results in detail (diagnose problems, understand proper inference) and will be confident to carry out the analysis and conclusions with respect to appropriatness and limitation of the methodology used. Finally, students will have sufficient grounding in econometric theory to begin advanced work in the field.
JEM059,061 - Financial Econometrics I and II (Masters)
The objective of the course is to introduce advanced time series methods. Students will be able to use the modern financial econometric tools after passing this course and will be prepared to continue in the Quantitative Finance II course. Part of the course is also focused on the high frequency data econometrics.
JEM116 - Applied Econometrics (Masters)
The course concentrates on the practical use of econometric methods, reviewing the relevant methodology, its use, and the possible alternative modeling approaches. The lectures are supplemented by computer classes, where students can gain hands-on experience in applied econometric analysis. During the course we will especially focus on time series techniques applied to forecasting asset volatility, modeling inflation, exchange rate volatility and other topics that you may regularly encounter in economic and financial literature. The course focuses on following topics in econometrics: OLS, IV, ARIMA, GARCH, VAR, cointegration, non-linear model and limited dependent variable. The course is especially suited for students undertaking empirical exercise in writing their M.A. thesis.
JED412,413 - Advanced Financial Econometrics (PhD seminar)
The aim of this seminar is an analysis of macroeconomic systems primarily by methods of nonlinear dynamics, stochastics dynamics, and nonlinear time series analysis. Special application will be focused on a behavior of the financial markets.
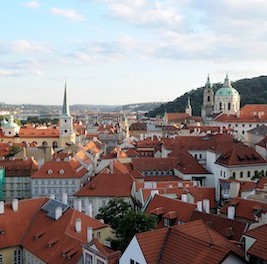
Contact
doc. PhDr. Jozef Baruník, Ph.D.
Associate Professor
Department of Macroeconomics and Econometrics
Institute of Economic Studies, Faculty of Social Sciences
Charles University
Opletalova 26
Prague 1, 110 00,Czech Republic
barunik [AT] fsv [DOT] cuni [DOT] cz
00 (420) 776 259273
https://ies.fsv.cuni.cz/en/contacts/people/29200599
Head of the Department
Department of Econometrics
Institute of Information Theory and Automation
Academy of Sciences of the Czech Republic
Pod Vodarenskou Vezi 4
Prague 8, 182 08,Czech Republic
barunik [AT] utia [DOT] cas [DOT] cz
00 (420) 776 259273
https://www.utia.cas.cz/people/barunik